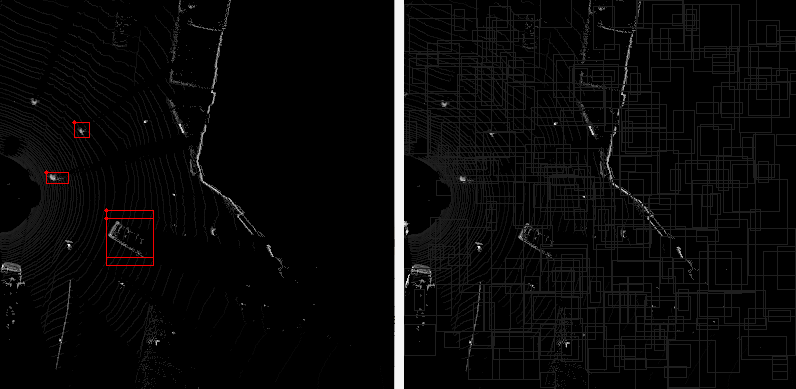
One of the big challenges with working on cutting-edge technology is the lack of established tools to rely on. Sometimes you have to build your own.
Here are tools that different Udacity Self-Driving Car students built to help them solve problems related to deep learning, computer vision, and the Didi Challenge!
Detecting road features
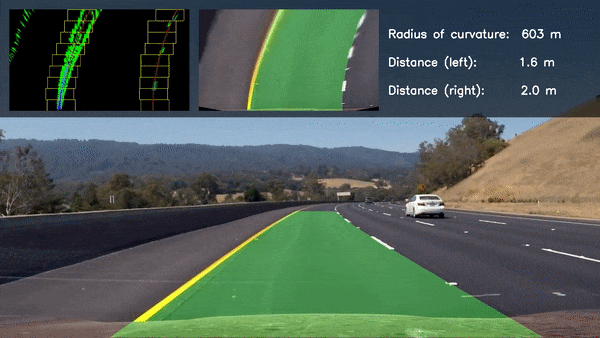
Alex provides great step-by-step analysis of his lane detection and vehicle tracking software. I really like his detailed explanation of the feature-tracking pipeline:
“After experimenting with various features I settled on a combination of HOG (Histogram of Oriented Gradients), spatial information and color channel histograms, all using YCbCr color space. Feature extraction is implemented as a context-preserving class (
FeatureExtractor
) to allow some pre-calculations for each frame. As some features take a lot of time to compute (looking at you, HOG), we only do that once for entire image and then return regions of it.”
Autonomous Vehicle Speed Estimation from dashboard cam
Jonathan built a really cool independent project to estimate vehicle speed from camera images. I really enjoyed his explanation of using optical flow for velocity:
“The Farneback method computes the Dense optical flow. That means it computes the optical flow from each pixel point in the current image to each pixel point in the next image.”
Transfer Learning in Keras
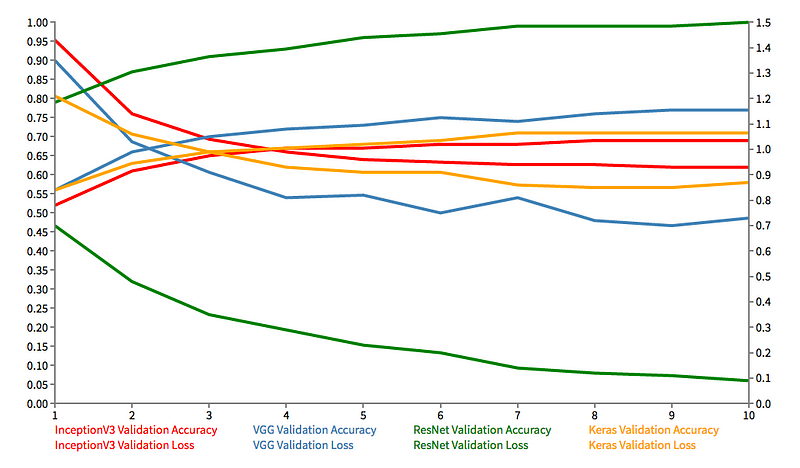
Galen is particularly interested in how to deploy neural networks in industry. To that end, he ran an experiment to see how well and how quickly various neural networks converged on classifying a training set:
“These networks (especially ResNet50 in this case) required extremely little training time and were relatively easy to implement. Once there is a proof of concept, it is a lot easier to write an optimized network that suits your needs (and maybe mimics the network you transfer learned from) than it is to both write and train from scratch.”
Attempting to Visualize a Convolutional Neural Network in Realtime
One of the knocks on neural networks is that they’re black boxes. Figuring out what drives their decisions is hard. Param built a tool to help visualize the internals of his network:
“On the right we have our Udacity Simulator running. On the left is my little React app that is visualizing all the outputs of the convolutional layers in my neural network.”
part.1: Didi Udacity Challenge 2017 — Car and pedestrian Detection using Lidar and RGB
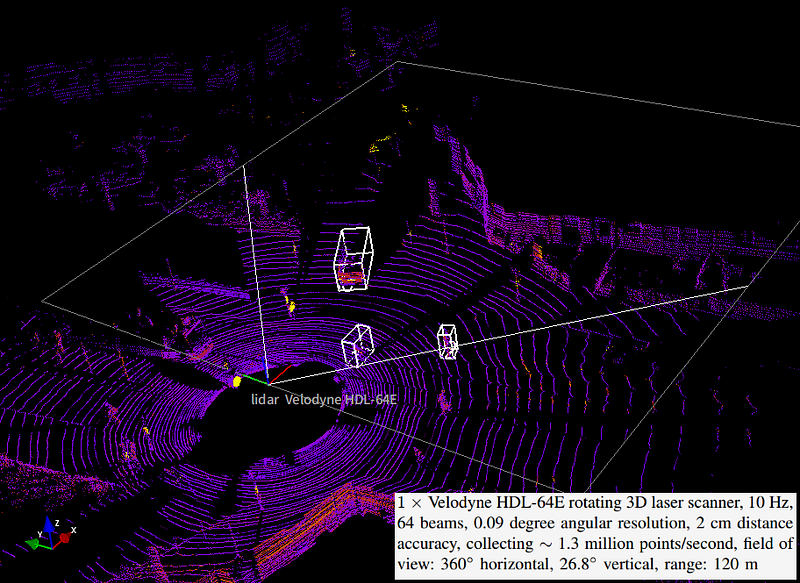
Cherkeng is keeping a diary of his work on the Didi Challenge!
“During development, visualization is very important. It helps to ensure that the code implementation and mathematical formulation are correct. I first covert a rectangular region of lidar 3d point cloud into a multi-channel top view image. I use the kitti dataset for my initial development”